What issue can we solve for you?
Type in your prompt above or try one of these suggestions
Suggested Prompt
A brief history of generative AI: timeline
2021: Generative AI tool DALL-E can generate images based on text input
2022: Conversational AI tool ChatGPT is released to the public
2023: Generative AI increases in complexity and capability with custom GPTs and third-party applications developed at scale
2024: Enterprise generative AI solutions are adopted organizationally at scale
Generative AI is rapidly evolving: Organizations should seize the business imperative now
Artificial intelligence (AI), machine learning (ML) and data science have become increasingly vital in the business landscape with generative AI recently entering the market.
It enables organizations to leverage data in a way previously not possible, streamline operational efficiencies at scales of magnitude and gain a competitive edge to meet customers where they are through personalization, develop new and creative approaches using content to engage and inspire, while breaking down organizational silos.
How can CEOs realize their vision for success with generative AI?
Teams developing business use cases for generative AI—while balancing the imperative for change—will require a CEO who is focused on developing a strategic mandate that is future-facing, while incorporating change management seamlessly and keeping pace with technological developments in the space. Here are six things CEOs should consider taking action on as generative AI takes hold in a competitive marketplace.

How to overcome prediction problems using enough data
In simple terms, an organization can only predict with any sort of confidence level if something is going to happen if there is a history of the action happening in the past. Trying to predict in areas with no precedence isn’t recommended, and leaders should make full use of any available data to drive decisions and solve problems using what is known, rather than what is thought to be known.
How much data is needed for generative AI to solve problems at scale?
So, is there a standard rule for how much data is needed? Data scientists say the more the better. An effective enterprise data strategy is key to being able to acquire, govern and retain key information needed at an organizational level.
“The data should be representative of the problem you are trying to solve for. If the data is skewed, your predictions will be skewed, too. Data scientists will typically look for clean data sets that they have a high level of understanding to train a model on and utilize feedback loops where possible to deliver continuous learning and increased accuracy.”
Ian Peebles , Director of Business Development, AI Labs
Generative AI has the power to overcome prediction problems by generating synthetic data that helps fill in the gaps where limited or incomplete data exists. Traditional predictive models rely heavily on historical data, which can often hinder accurate predictions when faced with complex or rapidly changing scenarios.
Generative AI, on the other hand, can generate data samples based on existing patterns, which can enable organizations to make better predictions—even in the absence of large datasets.

The difference between supervised and unsupervised learning for strategic development with generative AI
CEOs need to understand the distinction between supervised and unsupervised learning when it comes to generative AI. Generative models typically employ unsupervised learning to capture underlying patterns in the data, allowing them to generate new samples based on learned patterns. This acquired knowledge can help CEOs choose the right approach for their specific business needs, allowing their teams to capture the right data, for the right moment and the right time.
Supervised learning involves training the model using labeled data, whereas unsupervised learning extracts patterns and structures from unlabeled data.
“It is called ‘unsupervised’ because there is no predefined structure to the outcome. As an analogy, painting by numbers could be called ‘supervised,’ and some blank paper and colored pens ‘unsupervised.’"
Ian Peebles , Director of Business Development, AI Labs

How to use generative AI to identify appropriate areas of impact in business
Generative AI will have a profound impact on various areas of business. By understanding the potential areas of impact both present and future, CEOs can encourage leadership and their teams to leverage generative AI to improve their go-to-market advantage, whether it’s developing an operational model for anomaly detection or using data to augment revenue decisions. CEOs should be mindful that leaders secure buy-in with their teams so that levels of stakeholder interest and engagement are kept high as initiatives and use cases are identified.
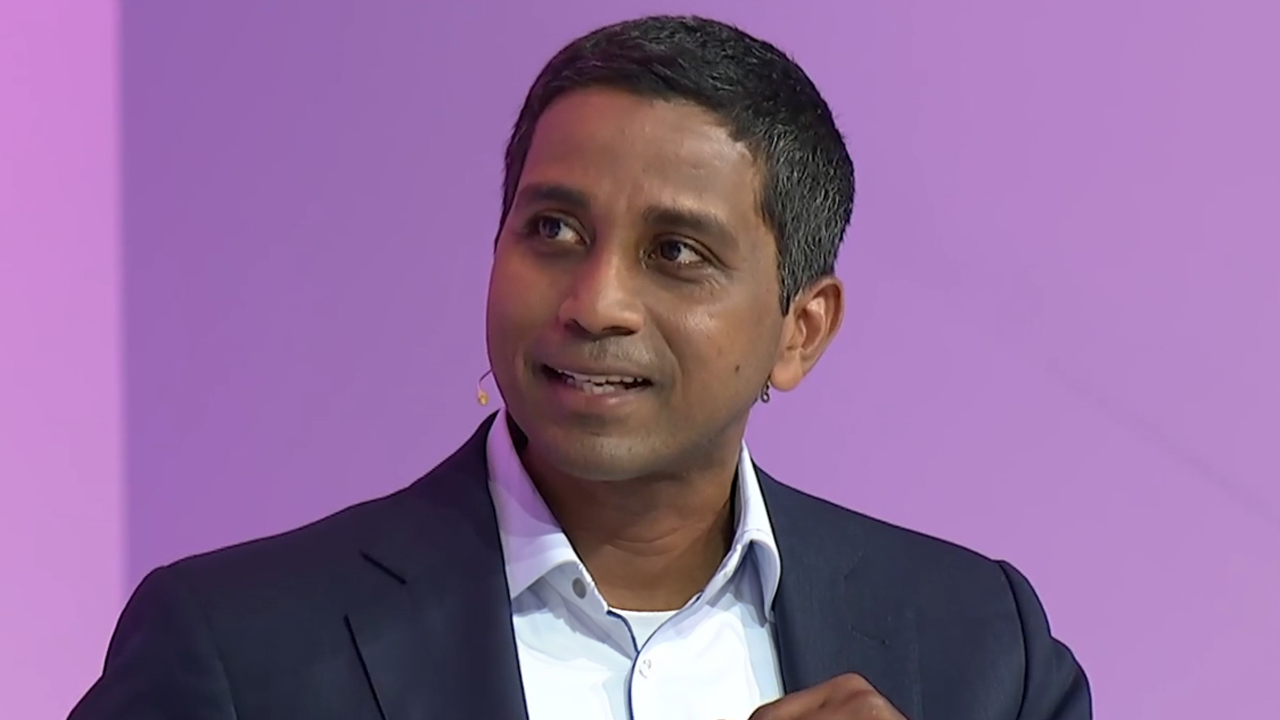

What neural and deep learning are and how they affect generative AI
Neural and deep learning are fundamental components of generative AI. While CEOs should have a basic understanding of the technology that plays into a top-down strategic vision, it’s expected that the drive to leverage this understanding will come from technology teams and data scientists that are best placed to make decisions on the adoption of specific generative AI technologies.
Neural networks are designed to mimic the human brain’s interconnected network of neurons, while deep learning refers to the training of neural networks with multiple layers to learn complex representations.
To process the data and all its variables, mathematical models are created. A model is sometimes also called an algorithm; machine learning uses many model approaches. The most common are:
- Linear, where the calculation tries to find the best fit for the data along a line.
- Tree-based, where possible outcomes are laid out in the form of a tree and its branches.
- Neural/deep learning, where more complex connections are laid out in layers, and the model learns about the relationship between the inputs to outputs.
Each of these model approaches have advantages and disadvantages depending on the data captured and the prediction problem that businesses are solving. These models typically calculate a confidence level in how accurate they think the prediction will be. As data becomes more trained, more accurate predictions can be gathered.

How to use generative AI to solve the right business problems at the right time
While generative AI can certainly present exciting opportunities, it is critical for CEOs and their teams to focus on solving the right business problems at the right time. Identifying opportunities that generative AI can address and aligning them with organizational goals requires executive leadership to inspire a vision for success with generative AI across the organization.
A relentless focus on solving the right problem with the right technology stack is key. Being able to prioritize speed of implementation versus go-to-market strategy are all considerations that should align with the organization’s policies, vision and goals.

How to handle the iterative nature of generative AI
When exploring generative AI, CEOs should plan for contingencies and embrace challenges as learning opportunities that can accelerate change and open new avenues of growth potential.
Having contingency plans in place will help mitigate potential risks and ensure the smooth integration of generative AI solutions into existing workflows. Common hurdles include:
- Unreliability/inexplicability of the model
- Regulation
- Existing processes or ways of working unable to effectively use new approach
- Lack of data
- Performance or scaling difficulty
- Unclear business case
Experimentation and trial and error are integral parts of adopting new technologies. By fostering a culture that encourages experimentation, CEOs can create an environment where “failures” are seen as stepping stones toward success.
“In practice, that means being prepared for failure and creating a culture of experimentation where organizations are testing hypotheses, rather than relying on the ways things are done today.”
Ian Peebles , Director of Business Development, AI Labs
The CEO’s imperative: how to lead the generative AI charge
CEOs play a critical role in understanding the nuances of generative AI and its future impact on their organization. Generative AI presents a transformative opportunity for organizations to gain a competitive edge, drive innovation and promote business growth.
By embracing generative AI with a strategic focus, CEOs can position their companies for scalable success in this era of AI-driven transformation.
Related Articles
-
Insight
Beyond Product Recommendations: How Retailers Can Increase Profits with Generative AI
Our experts summarize the state of generative artificial intelligence in retail in 2024, including use cases across customer experience, supply chain and back-end e-commerce.
-
Insight
What is Generative AI: From Now to Next
Generative AI is a type of artificial intelligence that is capable of creating new content, like images. How does it work, and how can business benefit?
-
Insight
How generative AI will revolutionize your business
Businesses can use generative AI to enhance workflows rather than replace human roles—with a more human-AI collaboration in mind.