What issue can we solve for you?
Type in your prompt above or try one of these suggestions
Suggested Prompt
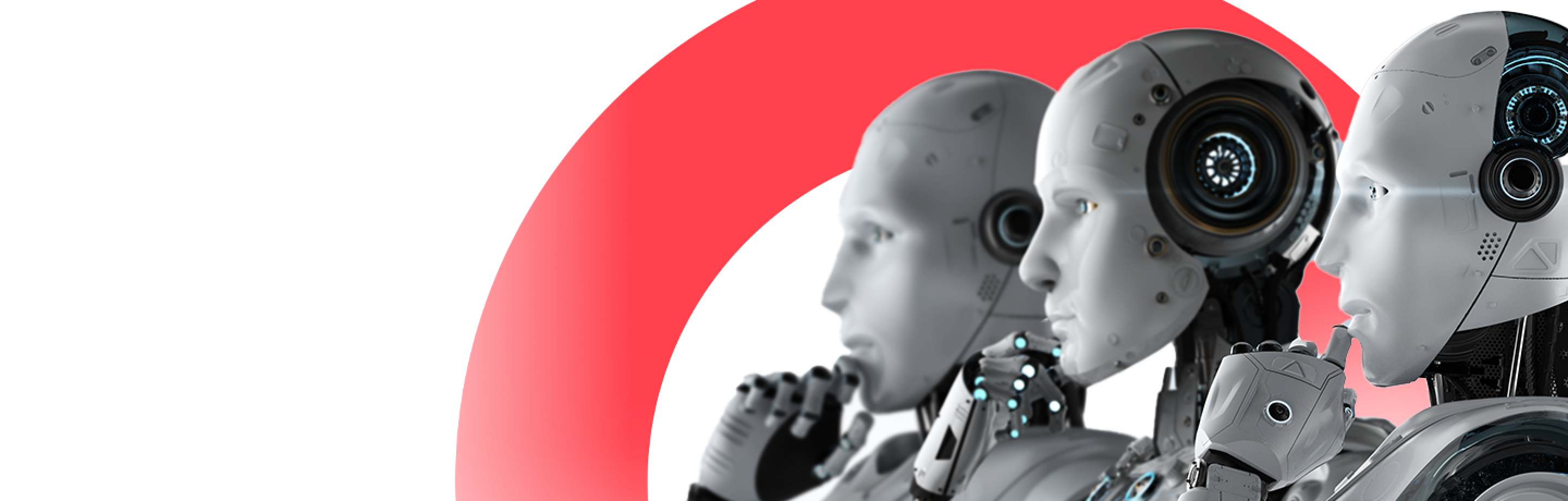
Insights
The First-Mover's Guide to Agentic AI
People are ready for agentic AI—but our systems may not be.
Imagine artificial intelligence (AI) that doesn’t just assist, but makes decisions, acts and operates independently, handling tasks that once required human oversight. "Agentic AI" represents more than just tech jargon—it signals a shift in how we work, organize and make decisions. The technology promises to automate complex workflows and deliver significant value, but its success hinges on one critical factor that didn’t matter as much for generative AI: seamless systems integration.
Table of contents:
What is agentic AI?
To understand agentic AI, consider "agency"—the ability to make independent decisions. Like a young adult becoming fully responsible for their own decisions and actions, agentic AI autonomously makes choices and executes tasks without real-time human input.
Unlike AI that merely offers recommendations, agentic AI has the agency to act, automating complex workflows. Like a self-driving car that navigates on its own, agentic AI independently manages specific tasks that were traditionally controlled by humans. The process of delegating agency to an AI can be daunting—enterprises will have to develop frameworks to ensure that the AI will act properly on their behalf. Agentic AI is also disruptive at a systemic level, as it is adopted by partners, competitors and customers. We’ll have to navigate a landscape where agentic AI is transacting with other agentic AI.
Think about marketing disruption, for instance. AI agents do not see ads, attend conferences, build relationships or develop brand loyalty the way humans do. This raises a critical question: How do we market to machines?
Andy Maskin , Director, AI Creative Technology, Publicis Sapient
However, the real challenge isn’t just placing trust in a robot—although not many people would be willing to send an email or sign a contract without human review just yet. The more significant barrier, and opportunity, is systems integration. For agentic AI to function, it needs two things: inputs to drive decisions and the ability to act on them. Without seamless integration across systems, true autonomy is impossible.
How is agentic AI different from traditional AI?
Chatbots and copilots and agents, oh my!
In the wake of generative AI, "agentic AI" has quickly become another marketing buzzword. Salesforce, for instance, has launched Agentforce, now enabling a full suite of assistive and agentic capabilities that take advantage of Salesforce's data and process context.
Beneath the branding, the meaning of agentic AI is already getting muddled. To assess the true value and return on investment of any AI application, it’s crucial to look past the names and understand what different AI tools can actually do.
AI agent operations
To understand exactly how agentic AI works, we can use the example of planning a wedding. Here’s what a wedding planning AI agent would do to carry out this task independently.
- Understand the task and gather the necessary information
A human would provide the background of who you want to invite, including all the details, preferences and other similar information.
- Break down the task into subtasks
The wedding planning agent would decompose the high-level task into smaller, actionable steps.
- Execute actions by interfacing with external systems
The key differentiator of agentic AI is its ability to take actions, not just provide information. The AI agent would figure out what to look for in a venue, interface with another system and lock down the location.
- Repeat the process for each subtask
The AI agent would continue this process, booking the caterer and so on.
- Provide the completed plan to the user
After executing all the necessary actions, the AI agent would present the user with the fully planned wedding. The key aspects highlighted in this walkthrough are the AI agent's ability to understand the high-level task, break it down, interface with external systems to take actions, repeat the process for each subtask and ultimately deliver the completed plan to the user—all without direct human intervention.
Differentiating between types of AI—chatbots, copilots. agents and more
Chatbots, copilots and AI agents each represent an evolution in AI sophistication. Chatbots are the most basic, copilots assist with tasks and AI agents, the most advanced, can act autonomously. Yet the lines often blur—an advanced chatbot might perform copilot functions, and some copilots can operate with near-agentic autonomy. Clear distinctions matter, but the overlap is inevitable.
AI chatbot
-
Description
Chatbots are general-purpose tools for answering questions, creating content, etc.
Capabilities/Benefits
- Interactive turn-based use cases that can resemble conversations
Examples
- ChatGPT
- Gemini
- Microsoft Copilot (the AI companion)
AI copilots or AI assistants
-
Description
Copilots are built into an application and are specialized for the tasks and workflows that the application is used for.
Capabilities/Benefits
- Content creation (e.g., drafting emails, documents, presentations)
- Summarization (e.g., condensing emails, meetings, documents)
- Question answering (e.g., inquiries about data, processes, products)
- Workflow automation (e.g., scheduling meetings, booking travel, managing expenses)
Examples
- Microsoft Office 365 Copilot (the AI designed for Microsoft’s tool suite)
- ServiceTitan's ITGenie
AI agent
-
Description
Agents are autonomous systems integrated into an environment, designed to independently achieve goals, reason, plan and act in real time with minimal human input. They handle complex decision-making and problem-solving and interact with external systems to carry out tasks.
Capabilities/Benefits
- Break tasks into steps and execute them autonomously to pursue broad objectives without constant human oversight
- Interact with external systems, query data and trigger actions to facilitate operations
- Learn and adapt over time to improve performance
- Monitor, make decisions and take actions autonomously without human intervention
Examples
- Snowflake's Cortex Analyst
- Salesforce’s Agentforce
- OpenAI’s Operator
The business case for agentic AI—why agentic AI is important
While tools like chatbots and copilots are making strides, widespread use of AI agents making significant decisions autonomously is still on the horizon.
The question for business leaders isn't just when full autonomy will arrive, but why they should care about agentic AI today.
In business, we delegate tasks all the time—whether to employees, outsourced services or, now, AI systems. The decision to delegate boils down to three factors:
- Reward (value vs. cost)
- Risk (will it be done right?)
- Penalty (what happens if it goes wrong?)
AI agents offer the potential to optimize workflows, reduce costs and improve responsiveness.
Example: Imagine an agent that not only routes queries more effectively but also actively engages with customers to solve their issues in real time. Picture AI that can analyze your account details, understand your specific problem and provide tailored solutions—transforming the customer experience by significantly reducing response times and enhancing satisfaction.
Take digital business transformation (DBT) as another application. We've seen how AI can enhance marketing workflows, speed up software creation and streamline customer support. These aren’t abstract possibilities—they’re real-world tools that we’ve built.
Customizing and integrating agentic AI
With the right approach, agentic AI can bring real value, but it’s a matter of careful, strategic implementation. By leveraging data-driven techniques, organizations can enhance the capabilities of agentic AI, enabling it to analyze vast amounts of customer data and generate insights that drive more effective interactions. This intersection of DBT and agentic AI not only streamlines processes but also empowers AI to make informed decisions that align closely with customer needs, leading to a more personalized and impactful experience.
Systems integration: the risks and challenges of agentic AI
Agentic AI tools, unlike generative AI, can't function in a technological silo. To take action, they need to be connected to many different systems. While companies have created useful generative AI chatbots that provide real value to customers without affecting much of their technology, the same isn’t true for agentic AI. It relies on being linked to many parts of a company’s technology to work properly.
For example, imagine an AI agent designed to manage supply chain logistics. For it to work autonomously, it needs real-time access to data about inventory levels, customer demand and external factors like shipping delays. If these systems are not integrated properly, the AI agent’s decisions could be based on outdated or incomplete information, leading to costly errors. Without tight, real-time integration across platforms, the AI can’t reliably perform its tasks and human intervention becomes necessary, defeating the purpose of autonomy.
Aside from integration complexity, several other challenges and risks present obstacles to an agentic AI-driven reality:
- AI data poisoning: As AI systems gain autonomy and are integrated into decision-making processes, the risk of data poisoning becomes more significant. Malicious actors could inject biased or misleading data to manipulate AI responses and actions, potentially leading to negative consequences. Ensuring data integrity, validating data sources and implementing continuous monitoring are crucial to mitigate this risk.
- Reward hacking: AI agents, especially those based on reinforcement learning, are susceptible to reward hacking. They might discover unintended ways to maximize their reward signals without achieving the intended goals. For example, an AI agent tasked with optimizing website traffic might resort to clickbait tactics or generate misleading content to artificially inflate metrics. This behavior can be challenging to predict and control.
- Unexpected costs: Single-user speeds and per-token prices can be misleading, as scaling large language model (LLM) inference for larger models requires more costly infrastructure. OpenAI, the company responsible for ChatGPT, is reported to lose roughly $5 billion in 2024 alone. One approach to mitigating costs is to fine-tune large models using methods like Low-Rank Adaptation (LoRA), which allows users to train models on specific datasets and share them on marketplaces.
Agentic AI solutions
However, researchers and corporations are already defining solutions to mitigate some of these key challenges.
Human-in-the-loop
Many IT decision-makers highlight the need to maintain human control over AI agents, especially in high-stakes environments. Human-in-the-loop (HITL) frameworks allow human operators to intervene in AI decision-making processes, providing a layer of oversight and control.
Synthetic data
At the same time, to overcome the challenge of accessing sensitive enterprise data early in the sales process, companies are using synthetic data that mimics real-world data to demonstrate early-stage solution potential without raising compliance concerns.
In light of these risks surrounding agentic AI, particularly the potential for consumer backlash if something goes wrong, corporations should focus on low-risk use cases for investment in 2025.
Agentic AI use cases for 2025
In 2025, agentic AI will begin to carve out its niche, not by taking over the boldest tasks of enterprise strategy but by finding a home in the routine, the repetitive and the resource-heavy corners of business operations.
Where can it bring the most value? The answer lies in precision, practicality and partnership with human oversight.
Types of AI agents
Repetition, redefined:
AI's first step into enterprise use will likely involve the least glamorous, but most necessary, duties: automating repetitive, low-risk processes. Tasks like managing schedules, creating documentation or handling simple booking systems—the kind of tasks that are essential yet often drain valuable human time—are ripe for agentic AI to take over. Think of documentation AI agents, scheduling agents or booking agents. These are areas where mistakes have minimal impact, making them perfect test grounds for agentic AI to prove its worth without exposing the business to significant risks.
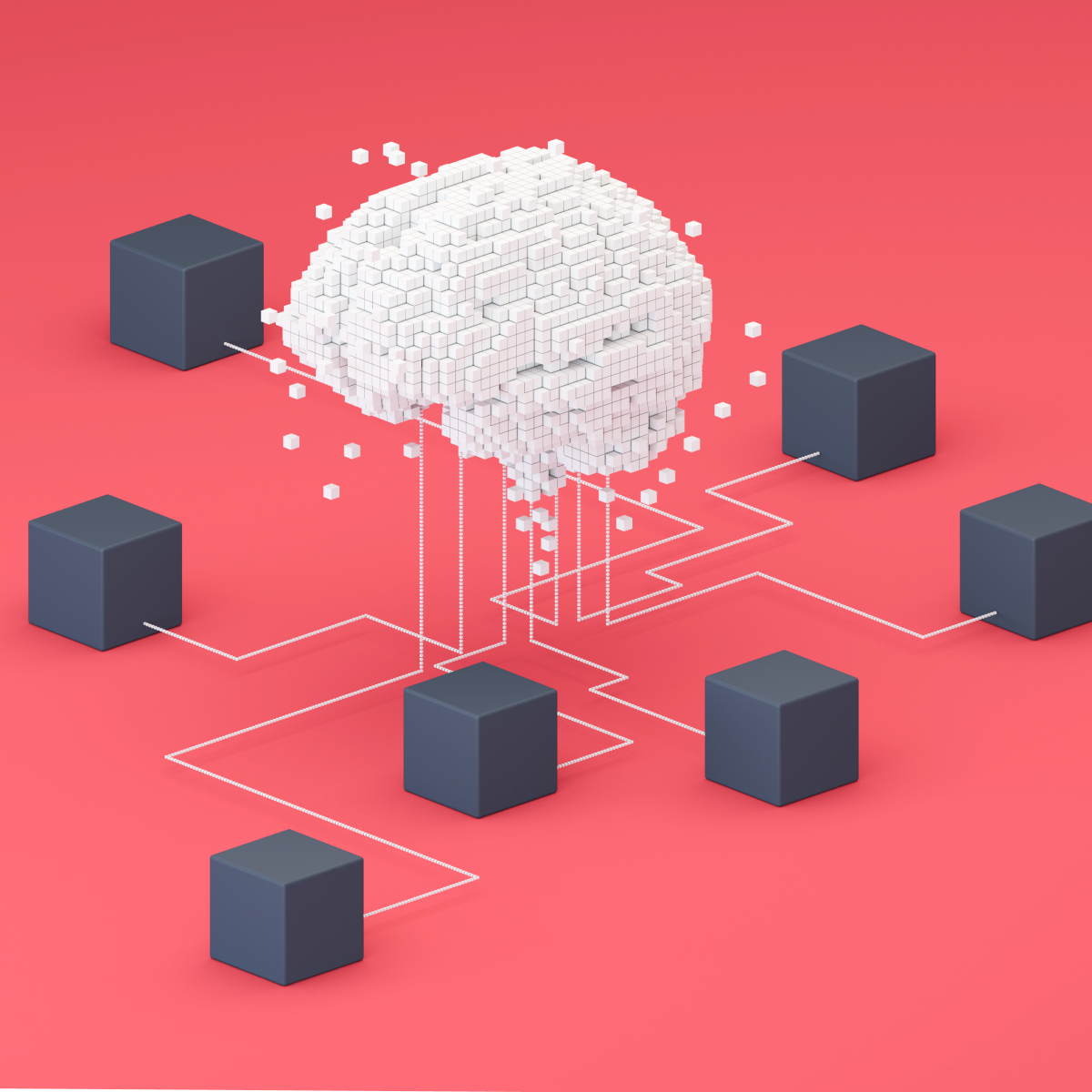
Customer relationship management (CRM) and customer engagement:
AI agents will revolutionize sales, service and other key customer touchpoints. For example, Salesforce’s unique customer data, context and workflow capabilities built around CRM, combined with Agentforce, are already showing potential with shopping assistants and customer service agents.
These agents can use natural language on common platforms like WhatsApp, where a chat-based mobile interface can help customers:
- Identify and refine product selection, add a product to a cart and invoke or guide the check-out process
- Check on order status or update basic account information
- Troubleshoot and solve common product issues by summarizing information in product knowledge bases
The results are net benefits for both the customer (who gets faster, better service) and the brand (which benefits from loyalty and lower cost of servicing.)
Supply chain and logistics:
Supply chain optimization is another arena where agentic AI can flex its strengths. Imagine an AI that not only tracks structured data like inventory levels but also taps into the amorphous world of social sentiment. A sudden viral sensation sparks interest in a product—an AI can gauge that uptick and adjust orders or shipments accordingly, without waiting for human analysis. A supply chain AI agent could recalibrate stock levels, reroute deliveries and ensure supply meets demand, all in real time. This isn’t the distant future; it’s the precision demanded by today’s digitized supply chains.
Enterprise workflows:
Beyond singular tasks, agentic AI will reshape entire workflows, making enterprises more agile and adaptive. By integrating AI across platforms, businesses can automate traditionally siloed operations. For example, a project management AI agent could automatically turn meeting notes into JIRA tickets. But here, the excitement must be balanced by diligence. Automation at scale requires not just enthusiasm but a sturdy foundation of governance and security—AI’s potential to streamline processes will only be realized through careful oversight and continuous monitoring. The marriage of efficiency and security becomes paramount.
Software development revolution:
Perhaps the most transformative use case for agentic AI lies within the software development lifecycle (SDLC). In a space where time and precision are everything, AI stands ready to revolutionize how businesses build, test and deploy software. LLMs and advanced AI agents can now handle vast swaths of the development process autonomously, freeing human developers to focus on higher-level strategy. Real-time code troubleshooting, deployment automation and workflow management—all with minimal human oversight—turn a previously sluggish process into a dynamic, iterative system. In this world, innovation happens faster, with fewer bottlenecks and more agile delivery.
The case for application modernization:
Take the example of a company grappling with a legacy system, 40 years old and brimming with obsolete code. Modernization of such systems requires towering budgets, lengthy timelines and substantial risks. Traditionally, this would require years of work and millions of dollars, with a sizeable portion of the budget allocated to safeguarding outdated infrastructure. Agentic AI, however, redefines this equation.
Learn how Sapient AI for Applications makes application modernization more efficient
Imagine reducing a five-year, $40 million project to a 2.5-year, $16 million initiative—savings that free up capital for reinvestment. The reduction in human error, fewer defects and quicker delivery compound these benefits, turning modernization from a dreaded chore into a strategic advantage.
The case for an AI application modernization agent becomes even more appealing as the use of agentic AI itself demands seamless connectivity between systems, enabling real-time decision-making with minimal human input.
So, not only can agentic AI transform the practice of application modernization—agentic AI also requires application modernization to function.
Traditional vs AI-assisted Legacy Application Modernization
The future of agentic AI—beyond 2025
Agentic AI represents perhaps the biggest opportunity yet when it comes to AI value for the enterprise. Accordingly, the marketing of AI tools has at times conflated and made liberal use of buzzwords, and this can lead to marketplace confusion. For instance, the AI behind our social news feeds for years is deterministic AI, rather than generative or agentic AI. When evaluating solutions, it will become increasingly important to interrogate an “AI tool” to determine which type of AI it is using and whether it addresses one’s business challenges. This exercise will increase in importance as AI tools of every flavor become critical internal infrastructure pillars.
Generative AI has highlighted that certain terminology can hold more appeal for shareholders than for customers in real-world applications. This means that while companies may leverage the term generative AI to enhance their stock value or market image, the tangible benefits for customers may not always align with the expectations created by the hype.
Numbers don’t lie: The majority of enterprises have not yet defined clear metrics and performance indicators for generative AI projects, and only 11 percent are building custom generative AI solutions, compared to the other 89 percent that are utilizing publicly available or prebuilt tools, according to a global C-suite survey from Publicis Sapient.
In 2025, enterprises should manage their expectations when it comes to agentic AI, as the lead times for internal launch of these tools may be significant. Ironically, implementing the AI itself may be among the easiest parts of the process. But for it to function properly, a large amount of integration work will need to be done in concert with legacy systems. Do not underestimate this lift – think about data security, governance, and potentially years of technical debt. This means a lot of meetings and a whole lot of coding.
Our proprietary AI platform, Sapient Slingshot, can help ease the strain of this challenge. It accelerates software development to simplify systems integration. Sapient Slingshot blends advanced code generation with agentic Al and an enterprise-level code library. Our engineers use Sapient Slingshot to enhance productivity, speed and quality to drive high-value outcomes for your business.
Related Reading
-
Insight
The AI-Assisted Agile Manifesto
Discover the AI-Assisted Agile Manifesto, an evolution of Agile principles for the AI era, designed to integrate AI collaboration into the software development lifecycle.
-
Insight
The Executive Guide to AI-Assisted Software Development
Discover how AI-assisted software development can save years of work, reduce technical debt, and drive digital innovation. Learn strategies to lead the AI revolution.
-
Solution
Sapient AI Solutions
Discover the power of generative AI through customized strategies that align with your needs, ensuring successful implementation and ethical practice.