What issue can we solve for you?
Type in your prompt above or try one of these suggestions
Suggested Prompt
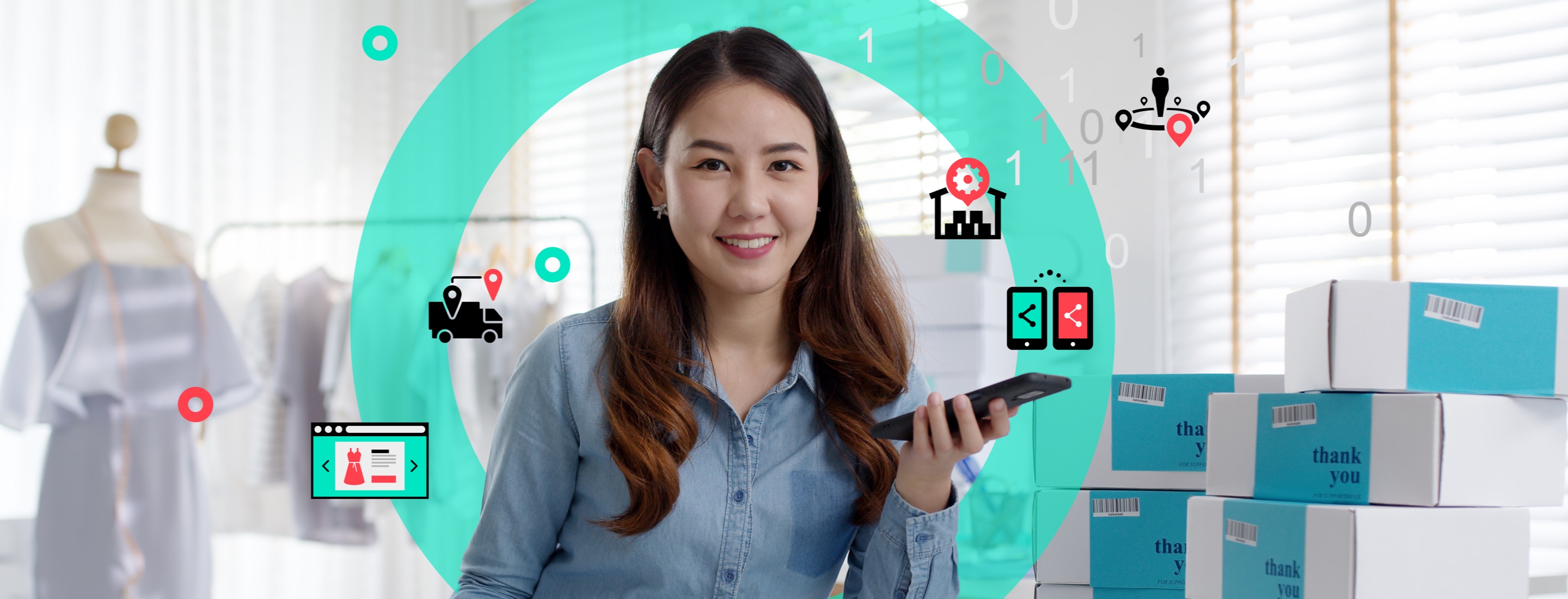
Insight
How can retailers grow their business through omnichannel fulfillment?
How can retailers grow their business through omnichannel fulfillment?
Put the right product in the right location at the right time and in the right amount to win customers
Retailers have been trying hard to adapt to rapidly changing consumer shopping behavior, especially in the last two years. They had no choice but to strengthen their omni-fulfillment capabilities to continue to serve customers through unprecedented times.
While some have been very successful, others have work to do. A study conducted by the MIT Center for Transportation & Logistics in September 2021 found several pain points that continue to pose challenges in the retailers’ omnichannel journey.
Here is a snapshot of these pain points:
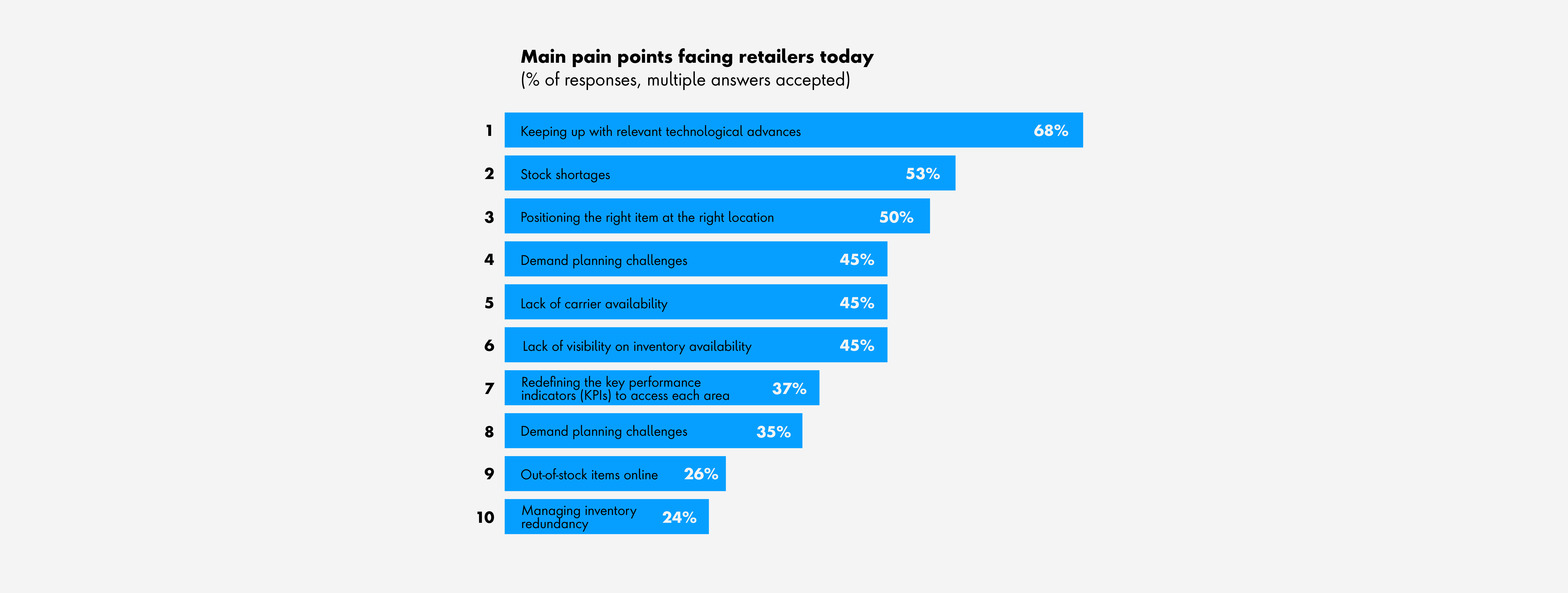
Source: Eva Ponce, “How Is Omnichannel Transforming Retailers’ Supply Chains,” Omnichannel Distribution Strategies Lab, MIT Center of Transportation & Logistics, 2021
Most of these pain points can be summarized in two broad issues:
1. Inventory projection
- Out-of-stock scenarios: Inventory not available to sell either due to under projection or incorrect timing (e.g., misalignment with demand)
- Inventory overage: Inventory procured more than what’s needed to satisfy the demand
2. Inventory placement
- Split shipments: Orders with more than one item could potentially ship from more than one fulfillment center
- Longer delivery times: The longer the distance between the customer ship-to location and the inventory location, the longer it takes to deliver the order to the customer
- Elevated shipping costs: Orders shipping from a farther fulfillment location result in higher shipping cost
- Higher inventory carrying cost: Inventory spread across a wider store network or placed in sub-optimal stores, resulting in higher inventory carrying cost
Addressing inventory projection issues
The key to successful inventory projection is a highly accurate demand forecasting process that uses past sales history, future growth, and other internal and external factors. These are a few factors to consider while implementing your demand forecasting solution:
Flexibility & Dynamism
Forecasting tools should be flexible enough to accept a wide range of input parameters. Sales history and future growth parameters are fundamental to the forecasting process. It’s a good idea to have at least two years of sales history for a meaningful demand projection. New product launches and new store launches must inherit the sales history from similar products and stores, respectively.
Future sales promotions, campaigns, discounts, and special events (concerts, sports events, tax-free weekend etc.) generally result in sudden spikes in demand and must be planned to make them successful. Additionally, seasonality, geographies, and location insights play an important role along with factors like economic and political circumstances – e.g., pandemic or support for green energy initiatives.
Real-time unplanned events (both internal and external) can have positive or negative impact on sales. A good forecasting tool should help your business to quickly react to these events and minimize the impact or maximize the revenue. This is possible if you can quickly adjust the input parameters, customize the models and features in real time, generate and regenerate the forecast as needed.
Accuracy
Demand forecasts are generally uncertain and inaccurate to start with. The key is improving the accuracy over time until the error rate falls within an acceptable threshold. Businesses can achieve this through benchmarking, performance measurement, ongoing optimization, and accurately interpreting sudden spikes in sales and dynamic scenarios due to unexpected events.
Insights and reports
Analytic insights are what fuel strategic business decisions about fulfillment. Several factors could be driving the future demand up or down. Some factors are significant and others, not so much. Timely and actionable insights into the drivers of these future demand fluctuations will help you proactively react by adjusting future inventory levels.
Business intelligence dashboards and reports allows users to foresee future out-of-stock scenarios and replenish the inventory quickly. Visualization of projected demand and supply levels via charts, tables, and gauges using interactive dashboards is key in maintaining optimum inventory levels. Especially when you can drill down to different levels of granularity - e.g., projection by region or by DC/store, by time, by fulfillment type (BOPIS, ship-from-store), by channel and by product hierarchy (division, department, class, sub-class, category, or SKU).
Scalability
As companies grow or diversify, the need for extending into additional regions, channels, markets, or product lines with minimal effort in configuring the forecasting process goes a long way and helps with speed to market.
Scalability to perform at a global level for those retailers looking to expand internationally may require additional considerations like lead times, local laws around product restrictions (e.g., country of origin), and local shopping patterns.
Artificial intelligence (AI) and machine learning (ML)
Technology helps retailers to understand customer behaviors and preferences and respond quickly and accordingly. Data-driven AI/ML-based forecasting has gained a lot of traction in the recent years.
This approach involves self-learning models that incrementally improve forecast accuracy based on feedback provided. One good example is consideration for real-time external factors such as commodity prices, income indicators, market performance, social media trends, etc. Machine learning algorithms can predict changes in consumer demand more precisely and can quickly recognize unusual demand patterns, complicated relationships in large datasets, and capture signals for demand fluctuation.
Predictive analytics provide insights into why a consumer is buying a product. This offers a deeper understanding of customer preferences and thereby gaining an edge over the competitors.
Addressing inventory placement issues
Retailers who have a significant physical store presence have expanded their fulfillment capabilities outside the traditional approach of shipping from a distribution center, by offering buy online pick up in store (BOPIS), ship from store (SFS) and ship to store fulfillment options. Many retailers are also exploring curbside pickup and same-day delivery.
These approaches expose the inventory across the distribution center and store network to online sales and the positioning of this inventory becomes extremely critical, considering increased customer expectations in the recent years.
So, the question keeping retail leadership up at night is how to bring the product closer to the customer so that his/her order can be fulfilled within a set of parameters that helps to keep the customers happy without hurting on the margins.
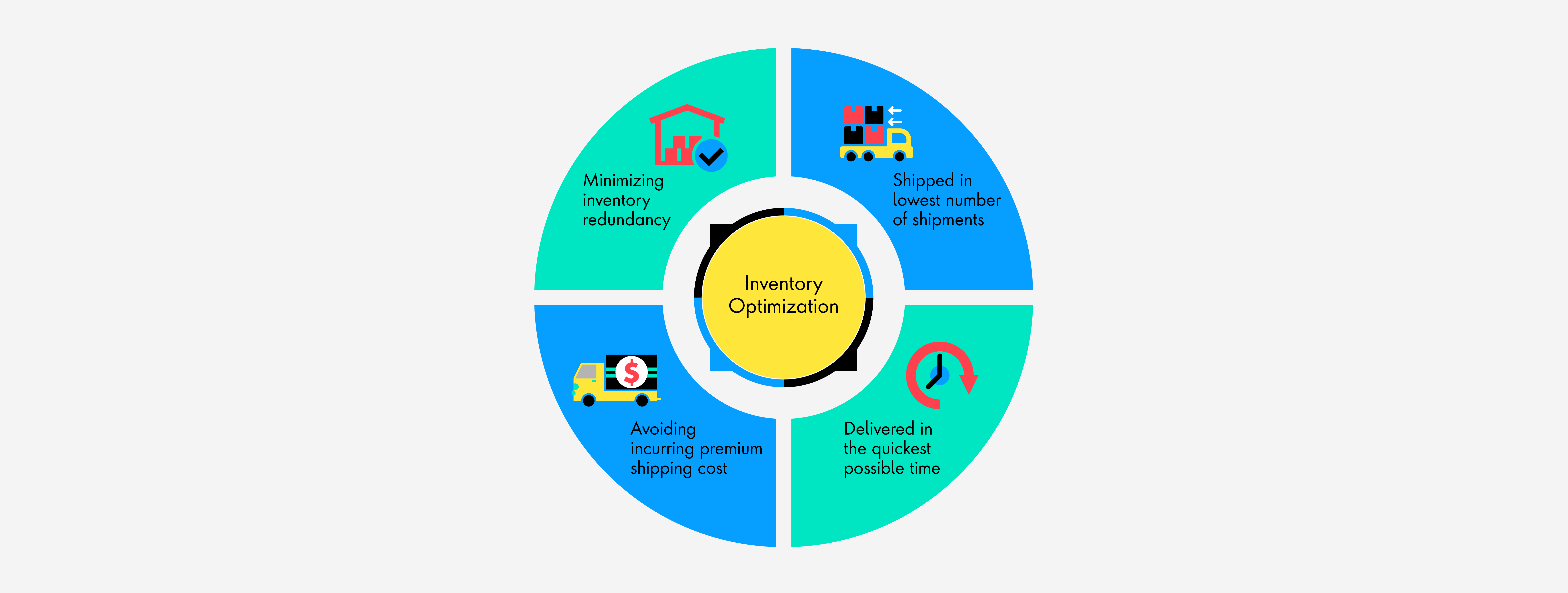
Fulfillment network strategy
BOPIS
- The fulfilment strategy for BOPIS is straightforward. Enable most of the stores (if not all) so that the customer has access to more choices.
SFS
- When it comes to SFS, the fulfilment strategy varies based on the network footprint, geography, and customer base. A retailer with 3,000 stores will have to adopt a different strategy than a retailer with less than 100 stores.
- For retailers with large number of stores, it may not be optimal to ship online orders from all their stores. Therefore, leaders can select a set of stores to perform this function and enable better control over inventory management and shipping operations.
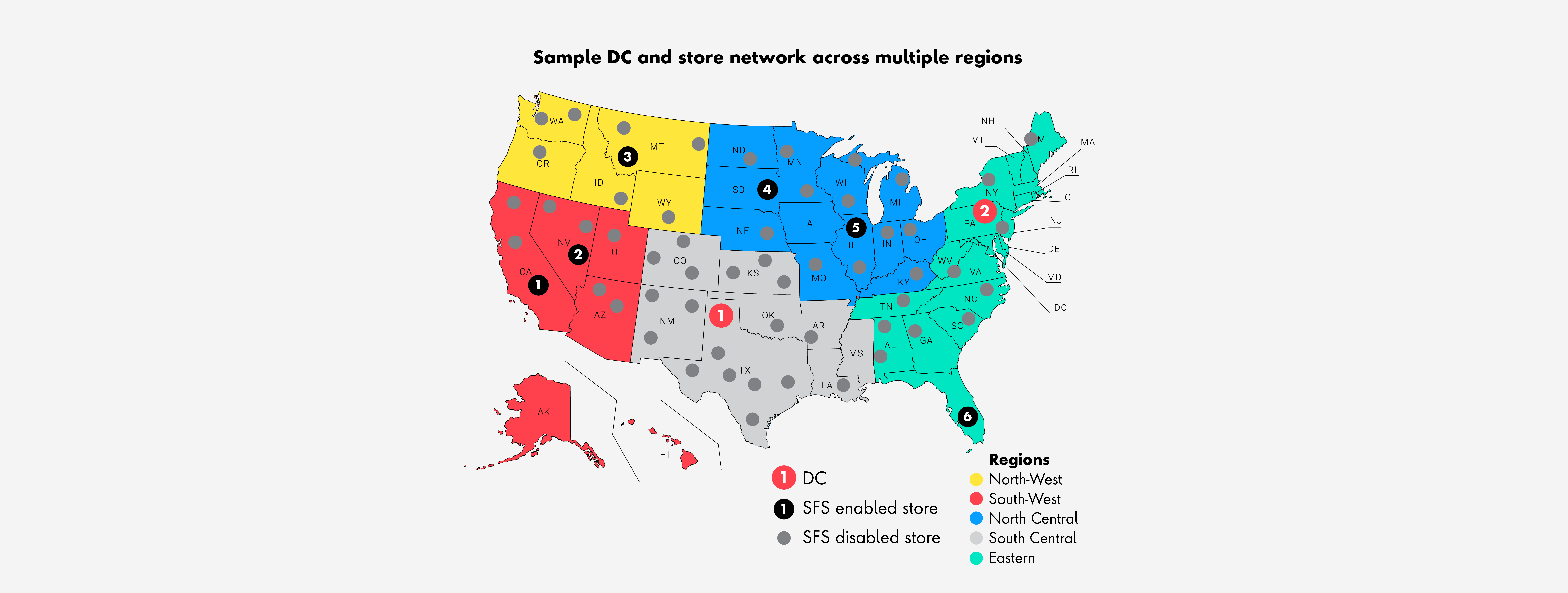
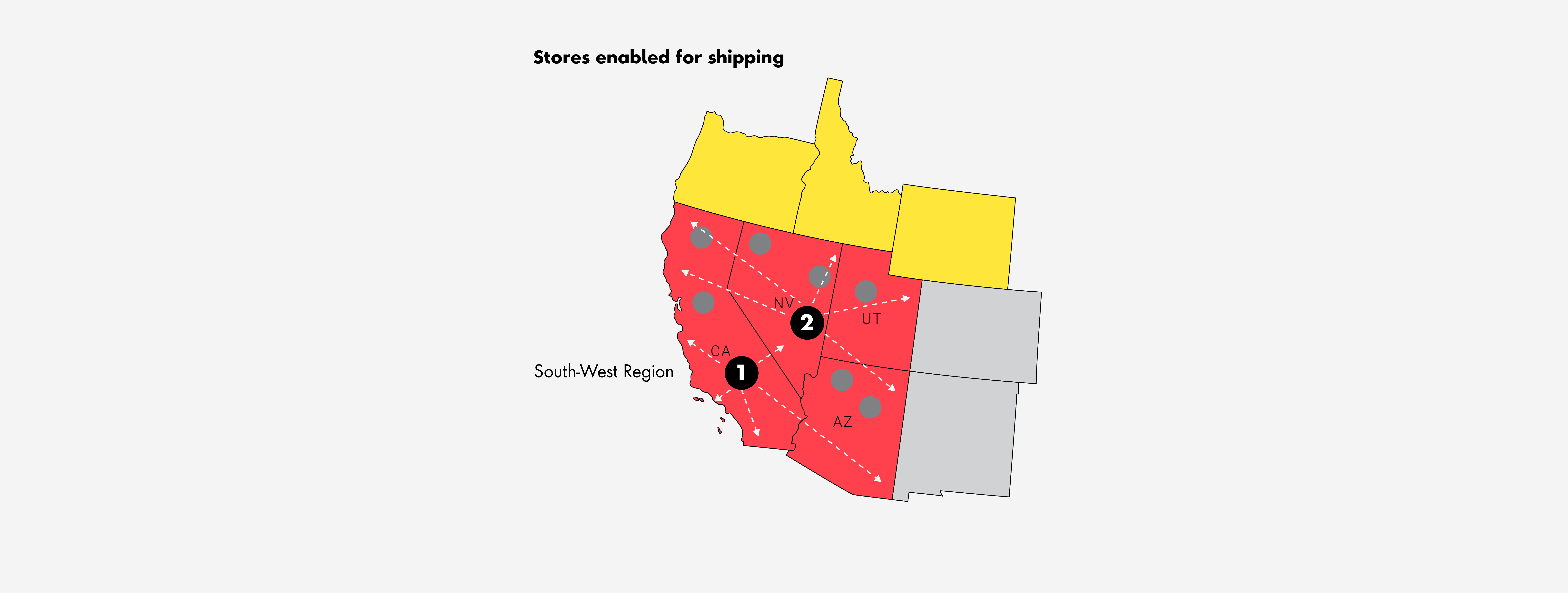
Key factors to consider in making a store SFS-enabled
-
SFS Stores Within a Region
Optimum number of SFS eligible stores within a region
Delivery SLA’s
All ZIP codes in a region within an acceptable standard shipping delivery SLA window from one of the SFS stores
Store Size and Capacity
Consider stores that have enough space to hold backroom inventory and capacity to run the fulfilment operations
-
Store With Heavy Foot Traffic
Exclude stores that have heavy foot traffic and/or the stores within busy ZIP codes
DC Proximity
Exclude stores that are in close proximity to a DC
Other Exclusions
Consider other exclusions that may be applicable (e.g., customer base, demand projections)
Inventory distribution strategy
The inventory procured to support the projected demand must be distributed across the fulfilment network (DCs and stores) based on several factors.
The size of the DCs and the backroom storage space in the stores forms the basis in determining the fulfilment network. In addition to storage capacity, storage requirements such as securing high-dollar items, firearms, or perishable items will help narrow down what stores/DCs can be made eligible to fulfill orders based on the product type.
Not all DCs/stores are equipped with the same level of operational capabilities. Having access to sufficient labor workforce, ability to handle large/fragile items or perishable products, ability to process regulated items such as medical devices/drugs, and ability to handle shipping are some of the factors to consider when enabling a store for SFS.
Product relationships and product assortments must be considered when placing products at a given DC/store. It is ideal to have the products from the same assortment be all collocated at a given location. For brands that offer value-added services, such as embroidery or monogramming, it is important to place products eligible for these services at a location that can perform these services.
Grow through omnichannel fulfillment
Today’s customers demand speed, convenience, and visibility into their orders more than ever. They want their orders fulfilled as promised with no delays or cancellations. Brands that meet customer demands have the greatest competitive advantage resulting in improved customer satisfaction and experience.
Accurate demand forecasting, combined with distributed inventory placement across the available DC and store network, may have the greatest impact in improving the speed of order fulfilment and delivery.
Retailers must find ways to bring the product closer to their customers and place them in strategic locations to achieve faster delivery at a reduced shipping cost. At the same time, they must minimize out-of-stock scenarios using robust demand forecasting processes and timely procurement. Lastly, it’s important to make products available to the customers when they need it and in the right quantities.
Satisfied, loyal customers help maintain and build brand value, which in turn, creates revenue growth.
Related Reading
-
Customer Data Platform: Switch on Your Sixth Sense
Publicis Sapient was born in the digital age, for the digital age. We offer customer-first insights and solutions to ensure our clients get the right CDP for their industry and individual challenges — fast.
-
Our Customer Data Platform (CDP) Quickstart Approach
This fast, deployable solution provides the cloud-native components to make it easier for companies to build an open source customer data platform (CDP). The tools are here to create a platform that enables data independence and customer centricity for everyone.
-
Building an Intelligence-Driven Consumer Products Firm
For CP firms, data transformation is about making better decisions that directly support critical business problems. This means establishing a process to capture, organize and analyze data across multiple sources to inform decision-making across the entire organization.