What issue can we solve for you?
Type in your prompt above or try one of these suggestions
Suggested Prompt
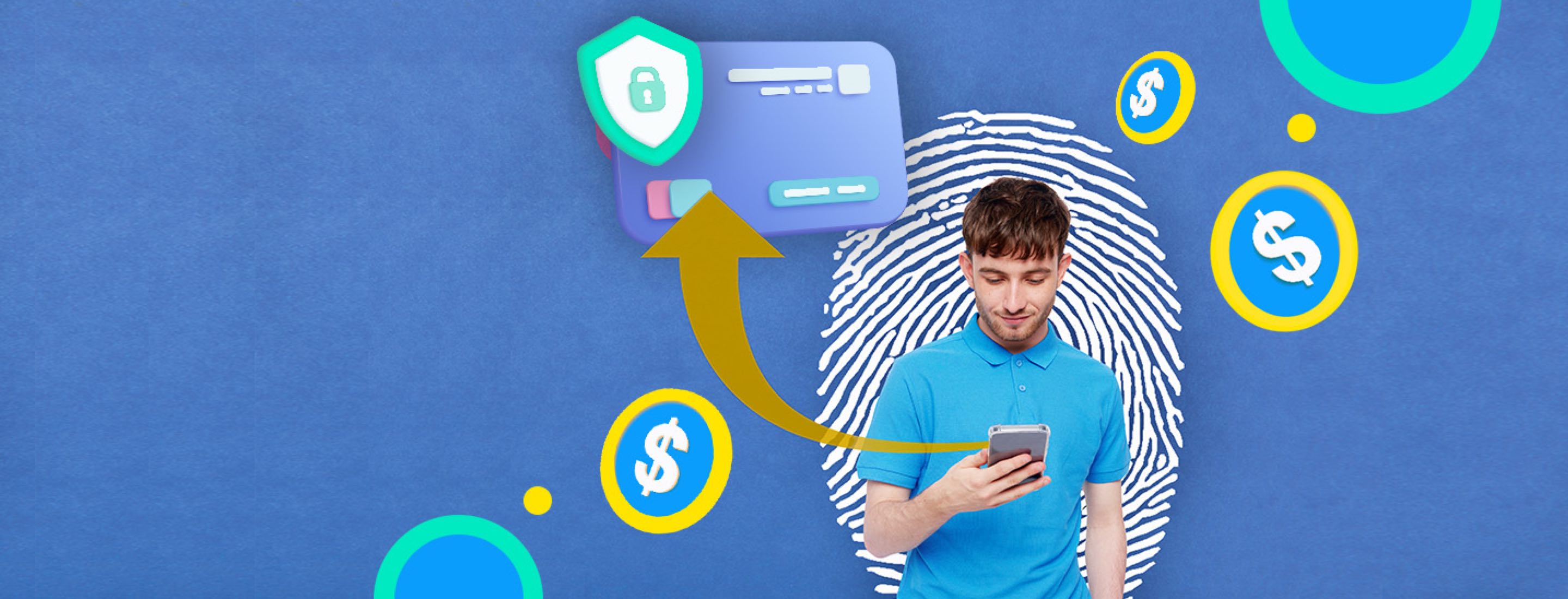
Travel & Hospitality
How to Create a Successful Fraud Anomaly Detection Program
How to Create a Successful Fraud Anomaly Detection Program
Is your brand’s loyalty program getting scammed by fake accounts? Learn the top challenges in preventing different types of mobile fraud for QSRs, hospitality brands and more, as well as the key strategies for implementing a fraud anomaly detection platform.
Every day, millions of offers are redeemed across restaurant chains, retailers and airlines through their mobile apps and loyalty programs. These offers aim to drive “incrementality,” such as an incremental increase in store visits or in bag size on an individual order.
However, with the increase in digital and especially mobile ordering and loyalty platforms, some customers have found ways to cheat the system through fraud and promotion abuse. This article will address which types of abuse are most prevalent, how fraud impacts bottom lines across industries, and the keys to success in creating fraud anomaly detection programs.
Types of mobile app and loyalty program fraud
These are the most common types of digital fraud:
Promotion abuse or new account fraud
In this case, the fraudster redeems multiple coupons through fake accounts using different email addresses, phone numbers or credit card numbers.
Account takeover (ATO) attacks
In this case, the fraudster redeems loyalty points through accounts that don’t belong to them, allowing them access to free food, merchandise or sign-on bonuses. With quick service restaurants (QSRs), fraudsters will perform “digital dine-and-dash” by reporting orders as incorrect or missing to get partial or full refunds.
Card testing
Card testing is when a fraudster uses a bot to test stolen credit card information through a series of small transactions, which can easily blend in with normal customer purchases. The action is often repeated before a brand or customer realizes the transactions’ fraudulent nature. Unfortunately, these actions cost businesses authorization fees between $0.15 and $0.25 per transaction, which rapidly add up.
How fraud anomaly detection prevents profit loss
On an individual basis, such activity might seem benign compared to an activity like bank account fraud. However, fraudulent actions are significantly impacting the bottom line for regional and global brands.
In one instance, college students scammed a total of $30,000 through repeated small transactions after discovering a glitch in a global dining brand’s mobile app. While the publicized nature of the scam sent the fraudsters to jail, if this trick were instead perpetrated through hundreds of isolated, separate customers, reimbursement would be difficult.
To put the financial loss in perspective, if a QSR experiences 60 fraudulent online chargebacks (CB) per month (or roughly 2 CBs a day) and that QSR has one hundred restaurants, the CB cost alone is $200k a month and over $2.4MM annually.
In another example, a major airline reported a trio of customers creating bogus loyalty program accounts to exploit millions of frequent-flyer miles. The fraudsters allegedly opened dozens of the airline's co-branded credit cards to earn sign-up bonuses intended for new users. While the dollar value of a mile is roughly one cent, this scheme alone cost the airline roughly $14,000.
Many brands account for promotional abuse and fraud in their accounting, or chalk it up to unavoidable consumer behavior. But as fraud becomes more sophisticated and frequent, brands need to implement anomaly detection platforms. These programs should leverage machine learning to look at different data signals, detect anomalous accounts and transactions, and take steps to address and prevent future fraudulent activity.
Isolation Forest (decision map) - blobs
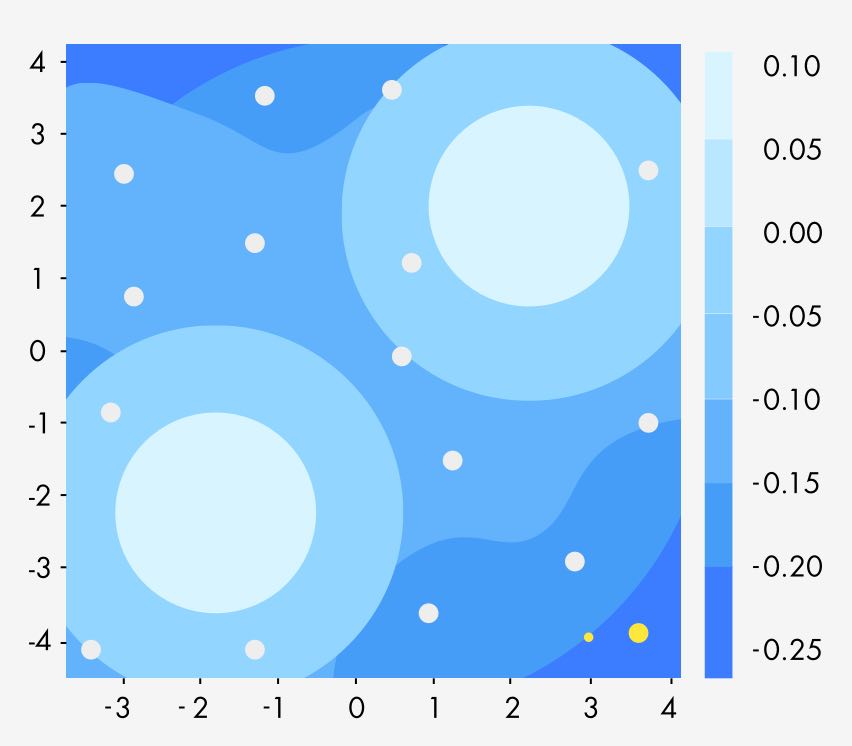
The top 5 challenges for mobile app and loyalty program fraud detection
While the reasons to implement a fraud anomaly detection program are clear, the steps often aren’t. One of the largest global QSR chains asked Publicis Sapient to create a platform to prevent and detect fraud cases such as coupon offer abuse, stolen loyalty point transactions and account takeovers. Through work creating this platform, Publicis Sapient discovered key barriers to fraud detection success, and how brands can overcome them.
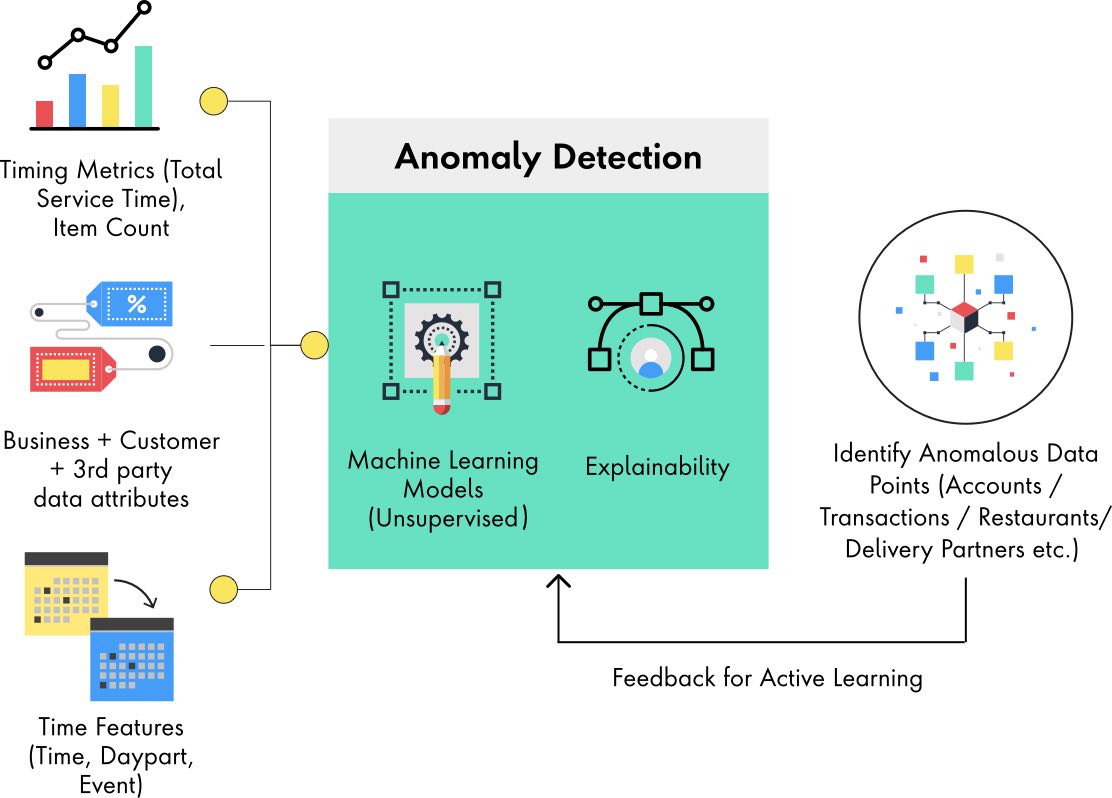
1. Fraud prevention is a never-ending journey
Brands should not assume that if they build an artificial intelligence (AI) platform, input all the customer and transaction data, and train a good machine learning (ML) model that fraudulent offer redemptions and transactions will quickly reveal themselves.
Fraud prevention requires more than a “build it and they will come” mindset; rather it requires a “catch me if you can'' mindset. In fact, since bad actors intend to blend in with legitimate users, fraud prevention is a journey that is continuously evolving. Fraudsters are always trying new methods to take over customer accounts, redeem unauthorized offers and more.
2. Risk signals are not black and white
One account may present legitimate-looking activity for a period of time before turning fraudulent, and potential risk signals blend in with positive traffic spikes.
Example: Consider Black Friday, when a wireless network operator might promote a BOGO for new phone lines. This event leads to a legitimate offer redemption traffic spike with many new lines added and dormant accounts coming to life. However, lurking underneath is also a higher level of fraudulent BOGO offers. It becomes challenging to accurately distinguish signal from noise, even for an ML model, which can lead to false positives and negatives.
3. Unpredictable variables allow fraud to fly under the radar
Because fraudsters often network together, when one discovers a new app loophole, it can lead to hundreds of rapid repeat attacks. New variables and scams are difficult for ML models to pick up fast enough, and oftentimes brands won’t be notified until days later.
Example: Two different loyalty miles redemptions from the same account occur milliseconds apart, but due to an edge case bug, they are both honored by the system, even though the points balance after the first transaction reaches zero. Effectively, the fraudster is getting more airline miles for free. If a larger group of fraudsters quickly and collectively operates hundreds of more accounts to exploit this same loophole, airlines may not notice. The unpredictability makes this problem highly dimensional and hard to model for accuracy.
4. Consequences for fraud are unclear and undefined
It is not sufficient to just discover fraud cases; there also needs to be a holistic operating model around how to deal with those cases. After the anomaly detection platform accurately predicts numerous instances of fraud, brands may find themselves at a place where the subsequent action is unclear and undefined.
Example: QSRs see cases of “friendly fraud,” where restaurant crew rack up points on their own loyalty accounts on behalf of customer purchases, i.e., customers receive a discount while the crew earns loyalty points on their own memberships. The decision process of whether to implement consequences, who orchestrates these consequences, and how the chain of action moves through the organizational machine is nebulous. Brands need to decide the outcome of fraud detection technology sooner rather than later to avoid delaying action after implementing the detection system.
5. Fraud detection can cause customer experience friction
Fraud protection and customer experience are often at odds, creating a dilemma for brand leaders. Blocking transactions in case of false positives or introducing additional authentication layers annoys and alienates good customers, while allowing fraud cases hurts margins.
Opinions on what actions to take in terms of user experience, functionality, fraud prevention and corrective actions will vary because of competing goals and KPIs. Getting initial alignment and revisiting processes with each new type of fraud is not simple, and there may not always be clarity on a path forward.
3 key strategies to implement successful fraud anomaly detection
Keeping in mind the importance of preventing mobile app fraud, how can leaders manage these challenges?
1. Create specialized fraud analytics capability teams
Some brands place fraud analysts within functional domain teams such as search, checkout, offers or payments. Given the complexity, variability, ambiguity and frequency of change happening in each area of fraud analytics, this model is difficult to scale. Fraud analytics, prevention and mitigation should be grouped into areas of specialization such as IP identification, address manipulation, account takeovers, malware attacks and more.
The benefit of adopting this alternative model is that it centralizes the people, processes, technology and data associated with each specific area of fraud analytics into distinct organizational units.
The strategy in practice: For example, the IP identification capability would specialize in improving accuracy of detection of fraudsters through IP analytics such as IP mismatches, repeat offender IPs, masked IPs, Tor IP proxies and more. All individual business domains such as account management, checkout and offers would leverage the expertise, services and tools of this IP identification capability team.
This specialized team is also responsible for both the strategy and execution of technology, data analysis, data privacy, and identification and combat of new threats related to all IP analytics—all while avoiding customer experience friction. Additionally, this team should introduce new fraud fighting tools, models and data enrichment associated with IP identification.
2. Apply site reliability engineering practices to fraud prevention
Brands should apply their existing site reliability engineering practices to fraud prevention, especially the concept of error budgets. Unlike typical software development, the activities of fraud prevention largely manifest themselves in the production environment. In many cases, production itself is the sandbox for fraud analysts to experiment and test and learn, and they need to be able to operate within a certain allowed error budget for both false positives and negatives.
If the fraud analysts are dinged every time fraudulent or abusive activity is not caught or prevented, then they will be highly conservative in their approach and push for measures that might introduce excessive friction in order to reduce fraud rates. If they are given room to fail and try out new tools and techniques, fraud rates may temporarily increase in the short term, but fraud detection accuracy will improve in the long term.
The strategy in practice: Using an error budget approach, brands can identify fraud prevention service-level objectives and KPIs like the fraud prevention rate. This ensures that the fraud prevention team meets service-level objectives under normal circumstances. Leaders can facilitate organizational commitment (for example: 5% for false positives and 8% for false negatives are allowed) for decision making and prioritizing investments.
Additionally, upper management needs to understand that error budgets are a key part of measuring the performance and impact of the fraud prevention team. Without alignment on these, it’s difficult to make material progress on improving fraud protection while reducing friction for the customer in a sustainable manner.
3. Define an operating model for fraud incident management
Finally, brands should define the fraud resolution operating model for valid fraud cases. At the very least, it should include a definition of the lifecycle of a fraud case and the departments that need to be involved, including digital product teams, customer service, store owner/operators and regional leads.
The strategy in practice: To ensure consistency across teams, leaders can outline processes for incident management, communication and collaboration, escalation management, retrospectives and continuous improvement. As the operation model comes to life in execution, a common language on how to communicate within the organization about fraud incidents and fraud protection should emerge.
Contact Publicis Sapient to learn more about fraud prevention solutions for digital organizations.
Related Articles
-
Case Study
Lufthansa Digitizes Customer Experience
For passengers of the leading German airline, mobile bag tag technology means no more waiting in line at the check-in counter.
-
How COVID-19 Shapes Digital for Restaurants
An Adobe and Publicis Sapient survey of quick-service and fast-casual restaurant executives looks at how they assess their transformation, how they have leveraged digital technologies and their digital maturity.
-
Return to Travel: What do Gen Z, Millennials and Gen X Expect?
Our global Travel & Hospitality report dissects the differences in travel preferences across generations.